A Sample of One?
IPR economist studies data collection for treatment choice
Get all our news
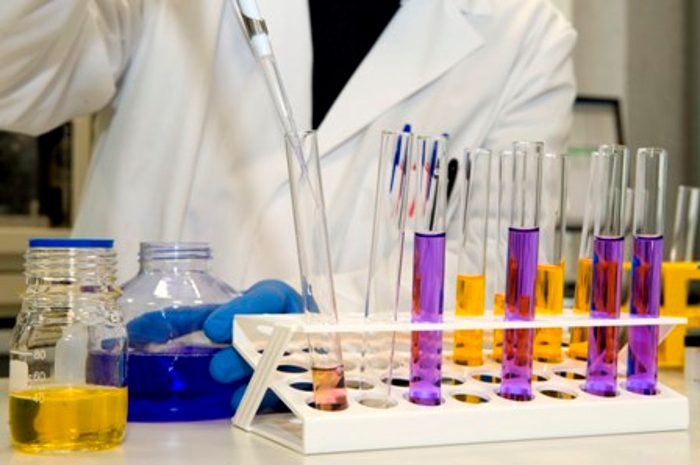
Can a randomized clinical trial be informative with a sample size of one per treatment? The answer is yes, according to new research published in the Proceedings of the National Academy of Sciences by IPR economist Charles F. Manski and Aleksey Tetenov, a former IPR graduate research assistant, now at the University of Bristol. The two challenge the use of hypothesis tests in medical decision-making, suggesting a way to reduce sample size in clinical trials and better inform treatment choice.
For researchers across disciplines, hypothesis testing, a statistical method first introduced in the 1920s, has become “ingrained,” especially in the field of medicine, Manski said.
He explained that we “often think of science as only progressing and overturning ideas, but instead a lot of times what happens is that something becomes the status quo.”
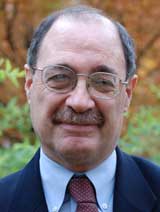
Today’s “status quo” clinical trials use hypothesis tests to determine whether a group of patients should receive an existing treatment or a new one. Under this method, “statistical power” determines what the sample size is by ensuring that a sample is large enough to suggest a certain level of confidence in the results.
But statistical power is “at most loosely connected to effective treatment choice,” the researchers wrote, making hypothesis tests unsuitable for informing clinical decisions. They also point to other well-documented drawbacks of hypothesis testing, which can only compare two treatments at a time, treats different types of statistical errors unequally, and fails to measure the impact when a clinician does not pick the best treatment. For Manski and Tetenov, helping clinicians choose the right treatment course is critical and what set them on the road to pursuing an alternative method in the first place.
Rather than relying on statistical power, their proposed method, rooted in statistical decision theory, uses near-optimal treatment rules to determine sample size. When a given treatment’s average effects are unknown—often the case in clinical settings—these near-optimal rules allow researchers to select treatments acceptably close to what they would have selected had they known the effects of each treatment.
Astonishingly, the researchers’ proposed method also drastically reduces the sample size needed to run an informative clinical trial. For instance, a sample size of 145 under near-optimal rules can be as accurate as a hypothesis test based on a sample of almost 8,000, Manski said.
And despite the paper’s focus on the clinical setting, Manski noted that these findings hold true across other fields as well.
“We wrote it up specifically in a medical setting, focusing on a medical case,” Manski said, “But everything we write in this paper would be applicable to a randomized experiment run by an economist.”
Charles F. Manski is the Board of Trustees Professor in Economics and an IPR fellow. The article, “Sufficient Trial Size to Inform Clinical Practice,” can be found here.
Published: September 29, 2016.